Dendritic gated networks: A rapid and efficient learning rule for biological neural circuits
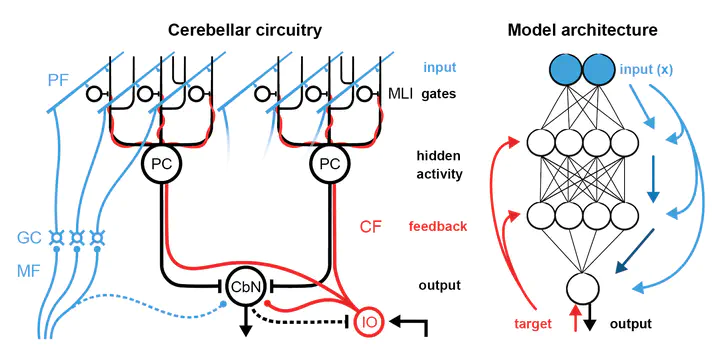
In this project, we introduce a powerful new biologically plausible machine learning algorithm: the Dendritic Gated Network (DGN), a variant of the Gated Linear Network. DGNs combine dendritic ‘gating’ (whereby ‘interneurons’ target dendrites to shape ’neuronal’ responses) with local learning rules to yield provably robust performance.
Importantly, DGNs have structural and functional similarities to cerebellar circuits, where (1) climbing fibers provide a well-defined feedback signal (Raymond and Medina, 2018), (2) the input-output transformation is relatively linear (Llinás and Sugimori, 1980; Walter and Khodakhah, 2006), and (3) molecular layer interneurons could act as local gates on learning (Callaway et al., 1995; Gaffield et al., 2018; Jörntell et al., 2010). To make this link explicit, we have performed two-photon calcium imaging experiments of Purkinje cell dendrites and molecular layer interneurons in awake mice. With these recordings, we find that single interneurons suppress activity in individual dendritic branches of Purkinje cells in vivo, validating a key feature of the model. Thus, our theoretical and experimental results draw a specific link between learning in DGNs and the functional architecture of the cerebellum. The generality of the DGN architecture should also allow this algorithm to be implemented in a range of networks in the mammalian brain, including the neocortex.
This project is a collaboration with researchers at the Gatsby Computational Neuroscience Unit at UCL and Google DeepMind.